A BENCHMARK FOR BUILDING INSTANCE SEGMENTATION IN SATELLITE IMAGES
In order to reach the goal of reliably solving Earth monitoring tasks, automated and efficient machine learning methods are necessary for scene analysis and interpretation. A typical bottleneck of supervised learning approaches is the availability of accurate (manually) labeled training data, which is particularly important to train state-of-the-art (deep) learning methods.
We present SemCity Toulouse, a publicly available, high-resolution, multi-spectral, multi-annotator benchmark data set for the training and evaluation of machine learning models. The benchmark acts as testbed for single building instance segmentation which has been rarely considered before in densely built urban areas. Additional information is provided in the form of a multi-class semantic segmentation annotation covering the same area plus an adjacent area 3 times larger. Moreover, the semantic segmentation stems from multiple annotators ranging from weakly to accurately labeled. The data set addresses interested researchers from various communities such as photogrammetry and remote sensing, but also computer vision and machine learning.
What do we provide?
- RGB and multi-spectral images covering the city of Toulouse, divided into 16 patches
- Semantic segmentations for all 16 patches, manually labeled by multiple annotators, with segmentation masks ranging from weakly labeled (annotator 1) to some labeling inaccuracies (annotator 3+4) to accurately labeled (annotator 2)
- Single-building instance segmentations for patches 3+4+7+8, manually labeled by professional assessors
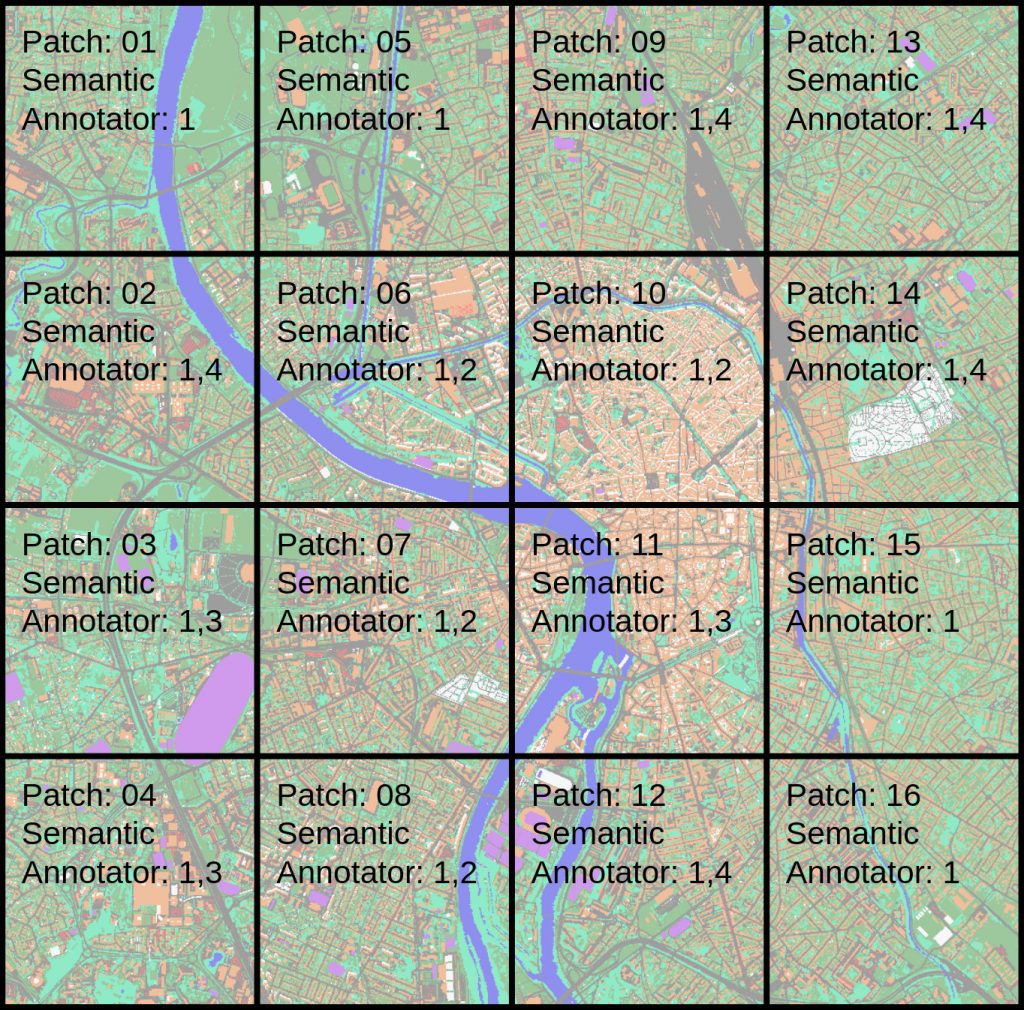
Please note: Updated semantic segmentations were added on 04/28/2022 without affecting the building instance segmentations. Therefore, the instance segmentation scores from the paper remain valid.
Download
If you are using the data please cite the following work
-
R. Roscher, M. Volpi, C. Mallet, L. Drees, and J. D. Wegner, “Semcity toulouse: a benchmark for building instance segmentation in satellite images,” Isprs annals of photogrammetry, remote sensing and spatial information sciences, vol. V-5-2020, p. 109–116, 2020.
[Bibtex]@Article{roscher2020semcity, AUTHOR = {Roscher, Ribana and Volpi, Michele and Mallet, Clément and Drees, Lukas and Wegner, Jan Dirk}, TITLE = {SemCity Toulouse: A Benchmark for Building Instance Segmentation in Satellite Images}, JOURNAL = {ISPRS Annals of Photogrammetry, Remote Sensing and Spatial Information Sciences}, VOLUME = {V-5-2020}, YEAR = {2020}, PAGES = {109--116}, URL = {https://www.isprs-ann-photogramm-remote-sens-spatial-inf-sci.net/V-5-2020/109/2020/}, DOI = {10.5194/isprs-annals-V-5-2020-109-2020}, Owner = {ribana}, Timestamp = {2020.05.06} }
People
Primary responsible
![]() | ![]() | ![]() | ![]() | |
Ribana Roscher | Michele Volpi | Clement Mallet | Jan Dirk Wegner |
Data and web page preparation / annotation refinement
![]() | ![]() | ![]() | ![]() | ![]() |
Lukas Drees | Johannes Leonhardt | Qusai Marashdeh | Verena Schiefer | Florian Sauerland |
Copyright
The datasets provided on this page are published under the Creative Commons Attribution-NonCommercial-ShareAlike 3.0 License. This means that you must attribute the work in the manner specified by the authors, you may not use this work for commercial purposes and if you alter, transform, or build upon this work, you may distribute the resulting work only under the same license. If you are interested in commercial usage you can contact us for further options.
