Welcome to the website of the Data Science for Crop Systems Group. Our research aims at the development of machine learning methods, which are particularly designed for the analysis of remote sensing data. We specifically focus on techniques for sophisticated feature learning and data analysis methods that integrate prior knowledge, such as scientific domain knowledge. We believe it is important to develop methods that ensure a high discrimination power and simultaneously model the underlying structure of the data. A particular research direction is explainable machine learning approaches which are able to tackle common challenges in the sciences such as the provision of reliable and scientific consistent results. These models give us a deeper understanding of what we have learned and can provide us with new scientific insights.
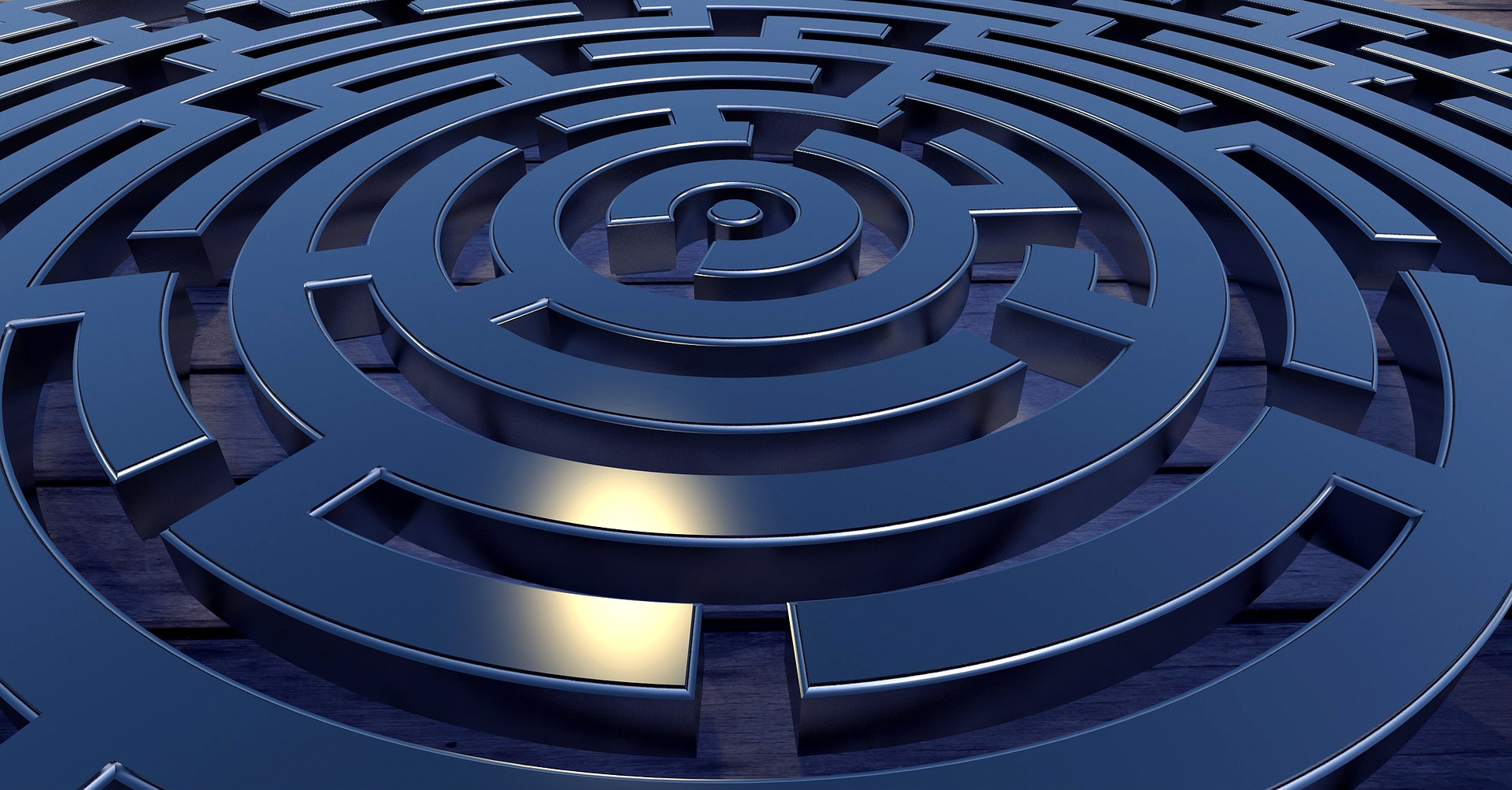
Open Positions
Right now, we don’t have open positions.